AI Research Agents: What Are They and Why They Are Taking Off
AI research agents are quietly reshaping how breakthroughs happen. Did you know that some AI agents can now generate, test, and refine hypotheses faster than entire research teams? That's not just efficiency; it's a paradigm shift.
Right now, we're at a tipping point. As research budgets tighten and the demand for innovation skyrockets, these agents are stepping in to fill the gap. But here's the twist: they're not just tools; they're collaborators, capable of offering insights that even seasoned experts might overlook.
And best of all : Drastically reducing the amount of time it takes to conduct knowledge research -- whether you are working on a tough research problem -- or a content writer researching a topic to write an in-depth article.
The Emergence of AI Research Agents
Here’s what’s fascinating:
AI research agents didn’t just appear overnight—they’re the result of converging advancements in natural language processing, machine learning, and data accessibility.
What sets them apart is their ability to reason through complex datasets, not just process them. For example, in drug discovery, AI agents like DeepMind’s AlphaFold have revolutionized protein structure prediction, solving problems that stumped researchers for decades.
In knowledge research, deep research agents like Gemini Deep Research and CustomGPT.ai Researcher are "doing the googling for you" and analyzing millions of words of knowledge in minutes.
But here’s the kicker: their success isn’t just about raw computational power. It’s about how they integrate with human workflows. By automating repetitive tasks like literature reviews or data cleaning, they free up researchers to focus on creative problem-solving. This synergy is where the real magic happens.
Still, adoption isn’t universal. Why? Factors like data quality, ethical concerns, and interpretability create barriers. Addressing these challenges requires a framework that prioritizes transparency, reproducibility, and human oversight—principles that could redefine how we approach innovation.
Defining AI Research Agents
AI research agents aren’t just tools—they’re adaptive systems designed to bridge the gap between raw data and actionable insights. What makes them unique is their ability to combine autonomy with collaboration. For instance, in climate modeling, these agents analyze vast datasets, simulate scenarios, and propose hypotheses, all while integrating seamlessly with human researchers to refine outcomes.
But let’s dig deeper. The real game-changer is their capacity for contextual reasoning. Unlike traditional algorithms, AI agents interpret data within specific research frameworks, ensuring relevance. This is critical in fields like personalized medicine, where agents tailor insights to individual patient profiles, driving breakthroughs in treatment.
Here’s what’s often overlooked: their success hinges on data diversity. Homogeneous datasets limit their potential, while diverse inputs unlock richer insights. Moving forward, researchers must prioritize inclusive data strategies and foster human-AI collaboration to fully realize their transformative potential.
Foundational Concepts of AI Research Agents
AI research agents are built on three pillars: autonomy, contextual intelligence, and collaborative adaptability. Think of them as the Swiss Army knives of research—they don’t just process data; they interpret, adapt, and act. For example, AlphaFold revolutionized protein folding by autonomously predicting 3D structures, a task that once took years of human effort.
But here’s what’s surprising: their power isn’t just in algorithms—it’s in how they learn. Unlike static models, these agents thrive on reinforcement learning, improving through trial and error. Picture a chess player refining strategies with every match; AI agents do the same, but across fields like drug discovery or climate science.
Now, let’s bust a myth. Many assume these agents replace researchers. Wrong. They augment human expertise, handling repetitive tasks while leaving creativity and judgment to us. The result? Faster breakthroughs, richer insights, and a future where humans and AI innovate together.
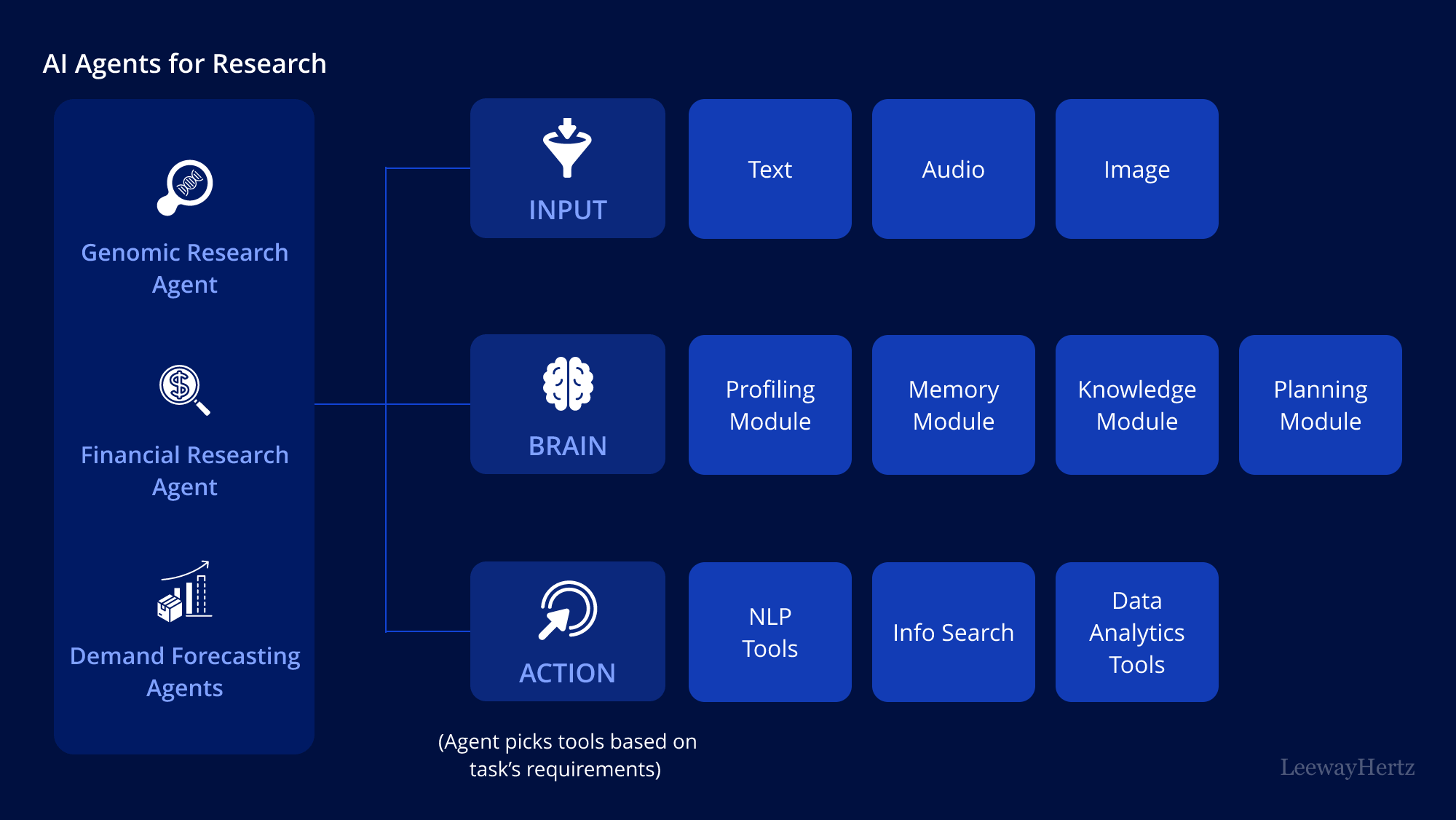
Image source: leewayhertz.com
Key Technologies and Algorithms
The backbone of AI research agents lies in transformer architectures like GPT. These models excel at natural language understanding by processing data in parallel, unlike traditional sequential methods. Why does this matter? Because it enables agents to analyze massive datasets—think millions of research papers—in seconds, uncovering patterns humans might miss.
For example, a single research task in CustomGPT.ai Researcher analyzes over 1.4M words within minutes.
But here’s where it gets interesting: multi-modal learning. By integrating text, images, and even sensor data, agents like DeepMind’s AlphaCode can tackle complex problems, such as generating code from natural language prompts. This cross-disciplinary capability bridges gaps between fields, fostering innovation.
One thing that has dramatically changed the trajectory of ai research agents is : COST -- the cost of powerful reasoning models like OpenAI's gpt-4o has great enhanced the ability to analyze and reason through lots of knowledge and data. This makes these AI research agents affordable and then consequently widely scalable.
Just a few month ago, analyzing 200 research papers would have cost over $20. Now that same analysis can be done for less than $1 -- vectorizing the entire content, or even conducting deep entity-relationship graph analysis on it (a technique called GraphRAG)
The Role of Generative AI in Research Agents
Generative AI is revolutionizing how research agents process and understand information. Unlike traditional models that simply classify or predict, GenAI creates new insights by understanding patterns and relationships across vast datasets. Think of it as the difference between a librarian who can find books (traditional AI) and a scholar who can synthesize new theories from multiple sources (GenAI).
Take CustomGPT.ai Researcher for instance. It doesn't just search through papers—it processes over 1.4 million words per research task, understanding context and nuance to generate comprehensive insights. This isn't just about volume; it's about comprehension at a human-like level.
But here's what most people miss: the real power of GenAI in research lies in its creative problem-solving. When analyzing drug interactions, for example, these systems don't just match known patterns—they can propose entirely new molecular combinations based on their understanding of chemical principles. It's like having a research assistant who not only knows the literature but can also generate novel hypotheses.
Here's the kicker: while older systems needed perfectly structured data, modern GenAI can work with messy, real-world information. Whether it's parsing through clinical notes, research papers, or social media discussions, these systems can extract meaningful insights from virtually any source. This versatility is why GenAI-powered research agents are becoming indispensable across industries, from pharmaceutical discovery to climate science to blog-post content writing.
Why AI Research Agents Are Gaining Momentum
AI research agents are thriving because they solve a fundamental problem — time. Traditional research workflows are bogged down by repetitive tasks like data cleaning and literature reviews. AI agents, however, automate these processes, slashing hours into minutes. For example, in drug discovery, AI systems like AlphaFold have predicted protein structures in weeks, a task that once took years.
But it’s not just about speed. These agents excel at connecting the dots. By analyzing diverse datasets—clinical trials, genomic data, and patient records—they uncover patterns humans might miss. Think of them as the ultimate research assistants, tirelessly synthesizing information across disciplines.
Here’s the twist: many assume AI replaces researchers. It doesn’t. Instead, it augments human creativity, enabling breakthroughs like personalized medicine. The takeaway? AI research agents aren’t just tools—they’re catalysts, transforming how we approach complex problems. And that’s why their adoption is skyrocketing.
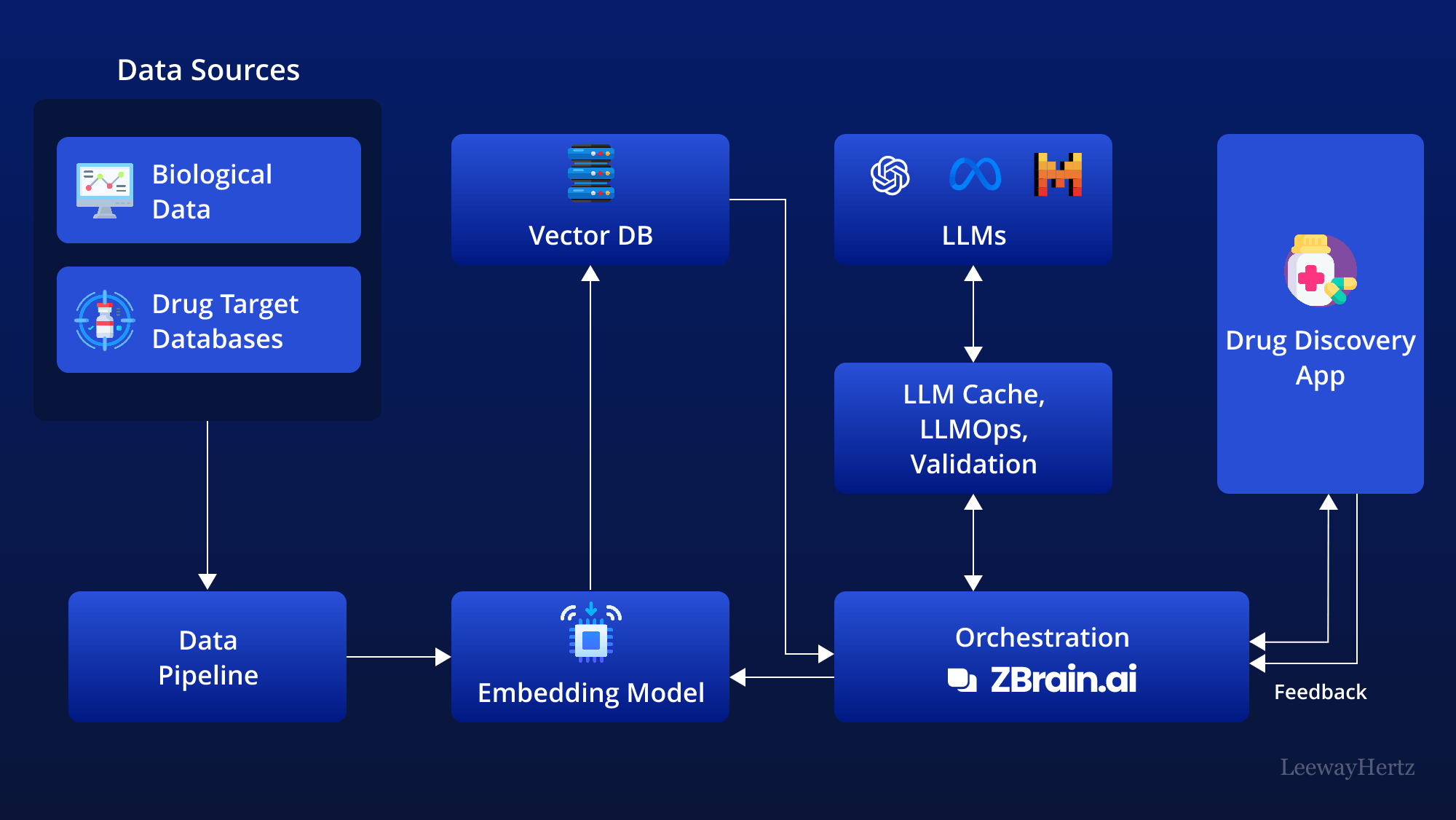
Image source: leewayhertz.com
Technological Advancements Driving Adoption
The real game-changer? Transformer architectures. Models like GPT and OpenAI's o1 have revolutionized how AI research agents process and understand data. Unlike traditional algorithms, these systems excel at contextual reasoning, enabling them to generate insights from unstructured data like scientific papers or clinical notes.
For instance, GPT-powered agents are now assisting researchers in drafting grant proposals by synthesizing relevant literature in minutes.
But there’s more. Multi-modal learning is pushing boundaries by integrating text, images, and even sensor data.
Imagine an AI agent analyzing MRI scans alongside patient histories to recommend personalized treatment plans. This cross-disciplinary capability is why industries like healthcare and manufacturing are adopting AI at breakneck speed.
Market Demand and Research Industry Trends
The surge in real-time data analysis and unstructured data is reshaping market demand. Research industries are no longer satisfied with static, retrospective insights.
AI research agents, leveraging live data streams from sources available via Google Search, are uncovering trends as they emerge. For example, in consumer goods, these agents predict shifts in purchasing behavior by analyzing sentiment data in real time—giving brands a competitive edge.
In my opinion, the biggest factor is the steep drop in cost associated with extremely powerful AI reasoning models like gpt-4o and o1. The cheap availaility of such high-reasoning intelligence is changing the research game.
Impact on Research Efficiency and Innovation
AI research agents are redefining hypothesis generation. Instead of relying on human intuition alone, these agents analyze vast datasets to propose hypotheses that might otherwise go unnoticed. For instance, in drug discovery, AI systems like AlphaFold predict protein structures, accelerating breakthroughs that would take years using traditional methods.
But there’s more. Cross-disciplinary insights are where the magic happens. AI agents synthesize data from unrelated fields—like combining climate science with epidemiology—to uncover novel connections. This approach has already led to innovative solutions, such as predicting disease outbreaks based on environmental changes.
In fact, one key technique (proposed by Stanford STORM) approaches knowledge research from a multi-perspective angle. So one can approach a topic with the thinking "Think like Einstein" or "Think like Feynman" and attack the topic from a different angle of curiosity. This approach has been adapted and refined by AI research agents like CustomGPT.ai Researcher.
Now, let’s challenge the norm. Many assume automation stifles creativity. Wrong. By handling tendious tasks, AI frees researchers to focus on high-level problem-solving. The actionable takeaway? Pair AI with human ingenuity and curiousity to foster a collaborative ecosystem where efficiency and innovation thrive.
Technical Implementation and Integration
Building AI research agents isn’t just about coding algorithms—it’s about creating a seamless ecosystem. Think of it like assembling a symphony orchestra: each component, from data pipelines to machine learning models, must harmonize. For example, CustomGPT.ai's Researcher utilizes different reasoning models and even GPT vision to analyze millions of words of knowledge. By having these models work in concert, one can generate excellent AI-based content and knowledge.
But integration doesn’t stop at technology. Human workflows are the missing piece. Remember: The output provided by the AI serves as a first-draft or knowledgebase for further refinement. This is NOT a tool to replace research. Just like Google did 20 years ago, these are tools to augment research and make it more efficient.
Now, let’s bust a myth: integration isn’t plug-and-play. It requires iterative refinement. Experts recommend starting small—like deploying agents for literature reviews—before scaling to complex tasks. The takeaway? Treat integration as a journey, not a destination, and prioritize adaptability over perfection.
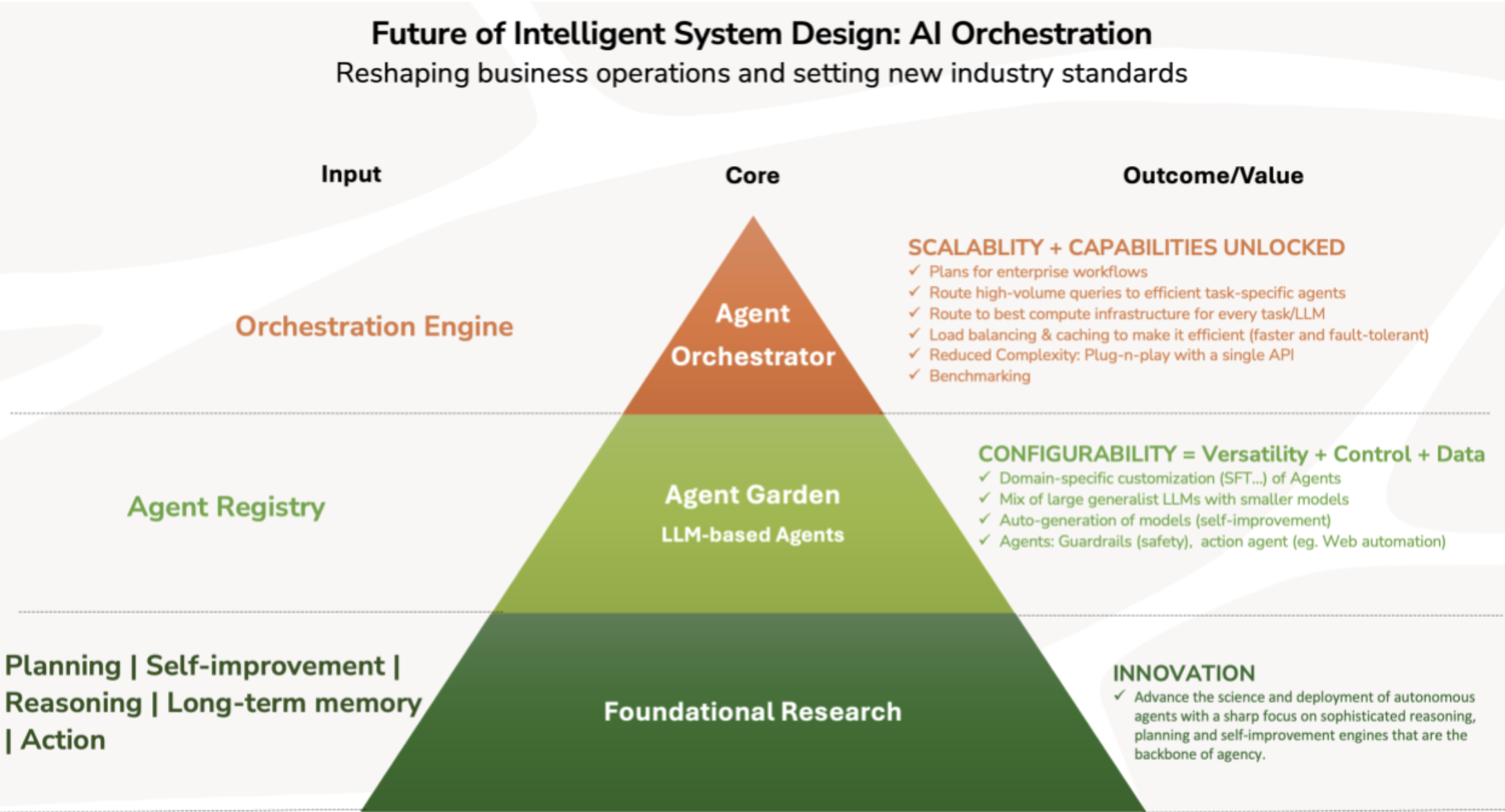
Image source: emergence.ai
Architectural Design of AI Research Agents
The architecture of an AI research agent isn’t just about stacking algorithms—it’s about designing a system that thinks, learns, and adapts. At its core, this involves three layers: input processing, cognitive reasoning, and output generation. For instance, OpenAI’s GPT models excel because their transformer architecture processes context across vast datasets, enabling nuanced understanding.
But here’s what most people miss: modularity is key. By decoupling components like data ingestion and hypothesis generation, researchers can swap or upgrade modules without overhauling the entire system.
Now, let’s connect the dots: this modularity mirrors principles in software engineering and even biology, where adaptable systems thrive. The takeaway? Build agents like ecosystems—flexible, scalable, and resilient. This approach not only enhances performance but also future-proofs your AI against evolving research demands.
Advanced Applications and Future Implications
AI research agents are redefining drug discovery. Take Insilico Medicine, for example. Their AI identified a novel drug candidate for fibrosis in just 46 days—a process that traditionally takes years. This isn’t just faster; it’s a paradigm shift, slashing costs and opening doors for rare disease treatments.
But it’s not all about speed. AI agents are also transforming climate modeling. By integrating satellite data with predictive algorithms, they’re uncovering microclimate patterns that were invisible to human analysis. Think of it as giving researchers a magnifying glass for the planet’s most pressing problems.
The future? It’s collaborative, not competitive.
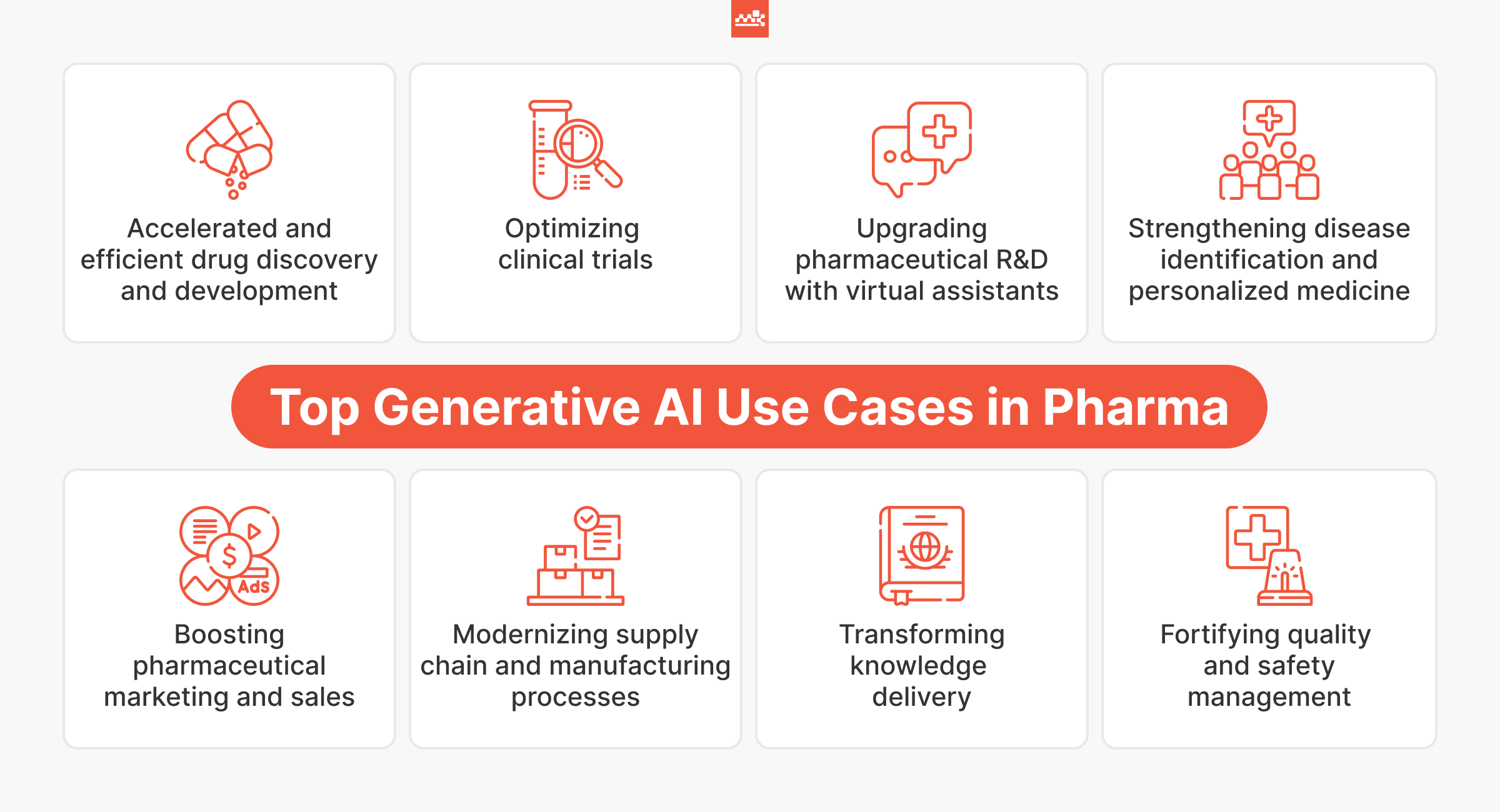
Image source: masterofcode.com
Case Studies in Scientific Discovery
AI research agents are revolutionizing genomic medicine. Take DeepVariant, an AI tool developed by Google. It deciphers genetic variations with 99.9% accuracy, outperforming traditional methods. Why does this matter? Because pinpointing mutations faster means earlier diagnoses for diseases like cancer and rare genetic disorders.
Now, let’s talk particle physics. At CERN, AI agents analyze petabytes of collision data to identify subatomic particles. This isn’t just about crunching numbers—it’s about uncovering the building blocks of the universe. By automating data filtering, researchers can focus on interpreting results, not sifting through noise.
But here’s the kicker: context matters. AI thrives when paired with domain-specific data. For example, combining AI with CRISPR research has accelerated gene-editing breakthroughs. The takeaway? Tailored datasets and interdisciplinary approaches aren’t optional—they’re essential.
For everyday people involved in research, or even knowledge research like Google Search, these systems represent a huge time-savings that drastically cut down the amount of time googling or pouring over large PDF documents.
Emerging Trends and Future Outlook
The rise of multi-agent systems (MAS) is reshaping how AI research agents operate. Unlike single-agent models, MAS enables multiple agents to collaborate or compete, simulating complex environments like traffic systems or supply chains.
Why does this matter? Because MAS mirrors real-world dynamics, making solutions more robust and scalable. In fact, even in research environments humans play different roles, and these multi-agents can simulate those roles like PI, researcher, reviewer, etc.
Take swarm intelligence, for example. Inspired by social insects, this approach allows agents to self-organize and adapt in real-time. It’s already being used in logistics, where fleets of autonomous drones coordinate deliveries efficiently. The key? Decentralized decision-making that reduces bottlenecks.
But here’s the catch: coordination costs. Without efficient communication protocols, MAS can become chaotic. The fix? Implementing game-theory-based strategies to optimize interactions.